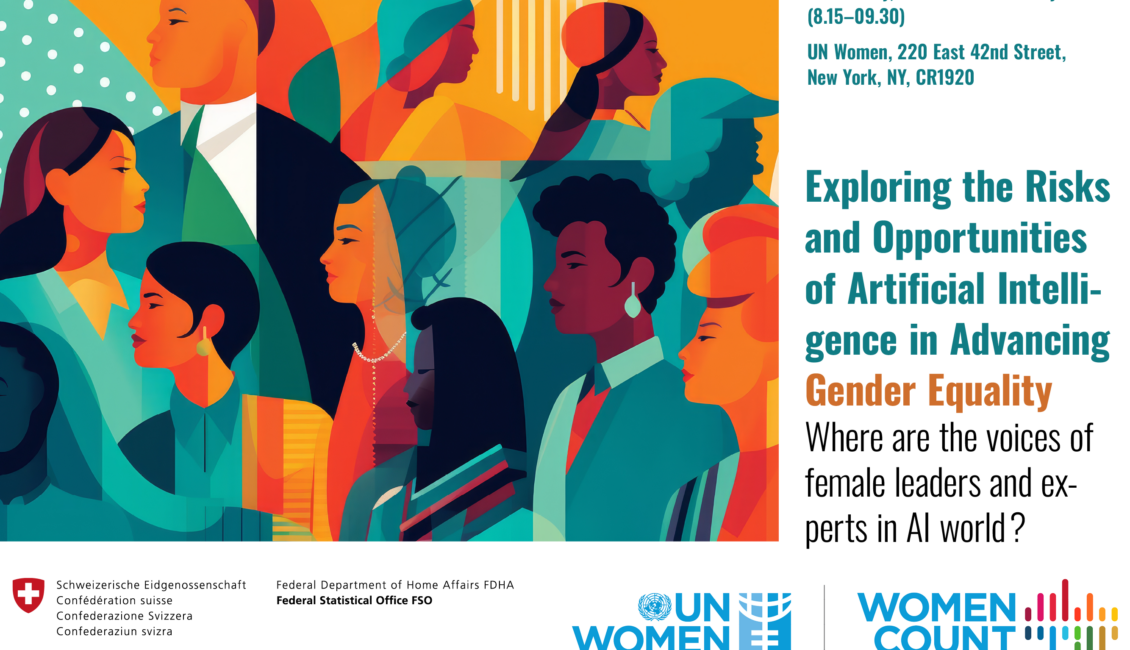
This event is organised by the Swiss Federal Statistical Office in collaboration with UN-Women.
Artificial Intelligence (AI) is rife with contradictions, while it has the potential to improve human existence, at the same time it threatens to deepen social divides. While AI tools do have the potential to improve diversity and inclusion, it doesn’t come from AI itself but rather from their creators. Indeed, AI appears neutral, but it’s made by humans, which means it internalizes all the same bias as we have – including gender bias. In addition, the problem stems from a lack of diversity within the industry that is reinforcing problematic gender stereotypes. Whether as developers, news editors or AI experts, women are largely absent from the AI world. Indeed, the systems are a reflection of broader gender disparities within the technology and A.I. sectors.
The side event will be the occasion to talk about opportunities AI offers but also the solutions we should take into account to lower gender gaps in the labor market as well as the gender representation within artificial intelligence thanks to better access to gender data. How can official statistics be aware of the data bias and how to take gender data into account while programming artificial intelligence? How can artificial intelligence transform gender gaps in the labor market and reduce gender inequalities?
Programme (see also flyer attached):
08:00-08:15 Welcoming breakfast
08:15-08:30 Key note speech : Eleonore Fournier-Tombs, Head of Anticipatory Action and Innovation, United Nations University (confirmed) – Centre for Policy Research and Ronald Jansen, Assistant Director of the United Nations Statistics Division (confirmed)
08:30-09:00 Moderated panel discussion with Georges-Simon Ulrich, Chief Statisticians of Switzerland and Vice-Chair of the UN Statistical Commission (confirmed); Tammy Glazer, Microsoft AI for good (confirmed); Sarah Steinberg, LinkedIn Global Public Policy lead (confirmed)
09:00-09:30 Q&A
This in-person event is by registration only, by 26 February 2024 at the latest. There are a limited number of places and it will not be possible to register at short notice. If you have any questions, please contact upd-initiative@bfs.admin.ch
Flyer
In the context of machine learning, bias can mean that there’s a greater level of error for certain demographic categories. Because there is no one root cause of this type of bias, there are numerous variables that researchers must take into account when developing and training machine-learning models, with factors that include an incomplete or skewed training dataset. Although, neutrality of primary data hardly exists in data nowadays. Models developed with this data can then fail to scale properly when applied to new data containing those missing categories. The aim to achieve gender equality and women’s empowerment runs throughout the UN’s Agenda 2030; it is a universal value alongside the commitment to leave no one behind. More specifically, SDG 5: Gender Equality also sets out to ‘achieve gender equality and empower all women and girls’ with concrete targets and indicators for every country.